
Titan Memory Server
Enables neural memory sequence learning with a memory-augmented model for improved code understanding and generation, featuring state management, novelty detection, and model persistence.
synthience
README
🧠 MCP - Titan Memory Server implementation
Colaboration between @jasonkneen and @ExpressionsBot
Follow us on X
An implementation inspired by Google Research's paper "Generative AI for Programming: A Common Task Framework". This server provides a neural memory system that can learn and predict sequences while maintaining state through a memory vector, following principles outlined in the research for improved code generation and understanding.
📚 Research Background
This implementation draws from the concepts presented in the Google Research paper (Muennighoff et al., 2024) which introduces a framework for evaluating and improving code generation models. The Titan Memory Server implements key concepts from the paper:
- Memory-augmented sequence learning
- Surprise metric for novelty detection
- Manifold optimization for stable learning
- State maintenance through memory vectors
These features align with the paper's goals of improving code understanding and generation through better memory and state management.
🚀 Features
- Neural memory model with configurable dimensions
- Sequence learning and prediction
- Surprise metric calculation
- Model persistence (save/load)
- Memory state management
- Full MCP tool integration
📦 Installation
# Install dependencies
npm install
# Build the project
npm run build
# Run tests
npm test
🛠️ Available MCP Tools
1. 🎯 init_model
Initialize the Titan Memory model with custom configuration.
{
inputDim?: number; // Input dimension (default: 64)
outputDim?: number; // Output/Memory dimension (default: 64)
}
2. 📚 train_step
Perform a single training step with current and next state vectors.
{
x_t: number[]; // Current state vector
x_next: number[]; // Next state vector
}
3. 🔄 forward_pass
Run a forward pass through the model with an input vector.
{
x: number[]; // Input vector
}
4. 💾 save_model
Save the model to a specified path.
{
path: string; // Path to save the model
}
5. 📂 load_model
Load the model from a specified path.
{
path: string; // Path to load the model from
}
6. ℹ️ get_status
Get current model status and configuration.
{} // No parameters required
7. 🔄 train_sequence
Train the model on a sequence of vectors.
{
sequence: number[][]; // Array of vectors to train on
}
🌟 Example Usage
// Initialize model
await callTool('init_model', { inputDim: 64, outputDim: 64 });
// Train on a sequence
const sequence = [
[1, 0, 0, /* ... */],
[0, 1, 0, /* ... */],
[0, 0, 1, /* ... */]
];
await callTool('train_sequence', { sequence });
// Run forward pass
const result = await callTool('forward_pass', {
x: [1, 0, 0, /* ... */]
});
🔧 Technical Details
- Built with TensorFlow.js for efficient tensor operations
- Uses manifold optimization for stable learning
- Implements surprise metric for novelty detection
- Memory management with proper tensor cleanup
- Type-safe implementation with TypeScript
- Comprehensive error handling
🧪 Testing
The project includes comprehensive tests covering:
- Model initialization and configuration
- Training and forward pass operations
- Memory state management
- Model persistence
- Edge cases and error handling
- Tensor cleanup and memory management
Run tests with:
npm test
🔍 Implementation Notes
- All tensor operations are wrapped in
tf.tidy()
for proper memory management - Implements proper error handling with detailed error messages
- Uses type-safe MCP tool definitions
- Maintains memory state between operations
- Handles floating-point precision issues with epsilon tolerance
📝 License
MIT License - feel free to use and modify as needed!
Recommended Servers
playwright-mcp
A Model Context Protocol server that enables LLMs to interact with web pages through structured accessibility snapshots without requiring vision models or screenshots.
Neon Database
MCP server for interacting with Neon Management API and databases
Qdrant Server
This repository is an example of how to create a MCP server for Qdrant, a vector search engine.
AIO-MCP Server
🚀 All-in-one MCP server with AI search, RAG, and multi-service integrations (GitLab/Jira/Confluence/YouTube) for AI-enhanced development workflows. Folk from
Persistent Knowledge Graph
An implementation of persistent memory for Claude using a local knowledge graph, allowing the AI to remember information about users across conversations with customizable storage location.
React MCP
react-mcp integrates with Claude Desktop, enabling the creation and modification of React apps based on user prompts
Atlassian Integration
Model Context Protocol (MCP) server for Atlassian Cloud products (Confluence and Jira). This integration is designed specifically for Atlassian Cloud instances and does not support Atlassian Server or Data Center deployments.
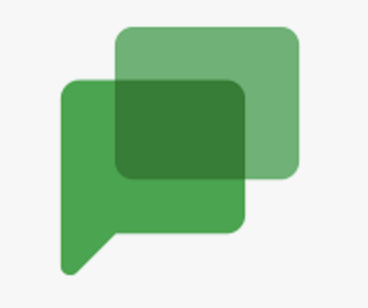
Any OpenAI Compatible API Integrations
Integrate Claude with Any OpenAI SDK Compatible Chat Completion API - OpenAI, Perplexity, Groq, xAI, PyroPrompts and more.
Exa MCP
A Model Context Protocol server that enables AI assistants like Claude to perform real-time web searches using the Exa AI Search API in a safe and controlled manner.
MySQL Server
Allows AI assistants to list tables, read data, and execute SQL queries through a controlled interface, making database exploration and analysis safer and more structured.