RAG Documentation
Enables AI assistants to enhance their responses with relevant documentation through a semantic vector search, offering tools for managing and processing documentation efficiently.
rahulretnan
README
RAG Documentation MCP Server
An MCP server implementation that provides tools for retrieving and processing documentation through vector search, enabling AI assistants to augment their responses with relevant documentation context.
Table of Contents
- Features
- Quick Start
- Docker Compose Setup
- Web Interface
- Configuration
- Acknowledgments
- Troubleshooting
Features
Tools
-
search_documentation
- Search through the documentation using vector search
- Returns relevant chunks of documentation with source information
-
list_sources
- List all available documentation sources
- Provides metadata about each source
-
extract_urls
- Extract URLs from text and check if they're already in the documentation
- Useful for preventing duplicate documentation
-
remove_documentation
- Remove documentation from a specific source
- Cleans up outdated or irrelevant documentation
-
list_queue
- List all items in the processing queue
- Shows status of pending documentation processing
-
run_queue
- Process all items in the queue
- Automatically adds new documentation to the vector store
-
clear_queue
- Clear all items from the processing queue
- Useful for resetting the system
-
add_documentation
- Add new documentation to the processing queue
- Supports various formats and sources
Quick Start
The RAG Documentation tool is designed for:
- Enhancing AI responses with relevant documentation
- Building documentation-aware AI assistants
- Creating context-aware tooling for developers
- Implementing semantic documentation search
- Augmenting existing knowledge bases
Docker Compose Setup
The project includes a docker-compose.yml
file for easy containerized deployment. To start the services:
docker-compose up -d
To stop the services:
docker-compose down
Web Interface
The system includes a web interface that can be accessed after starting the Docker Compose services:
- Open your browser and navigate to:
http://localhost:3030
- The interface provides:
- Real-time queue monitoring
- Documentation source management
- Search interface for testing queries
- System status and health checks
Configuration
Embeddings Configuration
The system uses Ollama as the default embedding provider for local embeddings generation, with OpenAI available as a fallback option. This setup prioritizes local processing while maintaining reliability through cloud-based fallback.
Environment Variables
EMBEDDING_PROVIDER
: Choose the primary embedding provider ('ollama' or 'openai', default: 'ollama')EMBEDDING_MODEL
: Specify the model to use (optional)- For OpenAI: defaults to 'text-embedding-3-small'
- For Ollama: defaults to 'nomic-embed-text'
OPENAI_API_KEY
: Required when using OpenAI as providerFALLBACK_PROVIDER
: Optional backup provider ('ollama' or 'openai')FALLBACK_MODEL
: Optional model for fallback provider
Cline Configuration
Add this to your cline_mcp_settings.json
:
{
"mcpServers": {
"rag-docs": {
"command": "node",
"args": ["/path/to/your/mcp-ragdocs/build/index.js"],
"env": {
"EMBEDDING_PROVIDER": "ollama", // default
"EMBEDDING_MODEL": "nomic-embed-text", // optional
"OPENAI_API_KEY": "your-api-key-here", // required for fallback
"FALLBACK_PROVIDER": "openai", // recommended for reliability
"FALLBACK_MODEL": "nomic-embed-text", // optional
"QDRANT_URL": "http://localhost:6333"
},
"disabled": false,
"autoApprove": [
"search_documentation",
"list_sources",
"extract_urls",
"remove_documentation",
"list_queue",
"run_queue",
"clear_queue",
"add_documentation"
]
}
}
}
Claude Desktop Configuration
Add this to your claude_desktop_config.json
:
{
"mcpServers": {
"rag-docs": {
"command": "node",
"args": ["/path/to/your/mcp-ragdocs/build/index.js"],
"env": {
"EMBEDDING_PROVIDER": "ollama", // default
"EMBEDDING_MODEL": "nomic-embed-text", // optional
"OPENAI_API_KEY": "your-api-key-here", // required for fallback
"FALLBACK_PROVIDER": "openai", // recommended for reliability
"FALLBACK_MODEL": "nomic-embed-text", // optional
"QDRANT_URL": "http://localhost:6333"
}
}
}
}
Default Configuration
The system uses Ollama by default for efficient local embedding generation. For optimal reliability:
- Install and run Ollama locally
- Configure OpenAI as fallback (recommended):
{ // Ollama is used by default, no need to specify EMBEDDING_PROVIDER "EMBEDDING_MODEL": "nomic-embed-text", // optional "FALLBACK_PROVIDER": "openai", "FALLBACK_MODEL": "text-embedding-3-small", "OPENAI_API_KEY": "your-api-key-here" }
This configuration ensures:
- Fast, local embedding generation with Ollama
- Automatic fallback to OpenAI if Ollama fails
- No external API calls unless necessary
Note: The system will automatically use the appropriate vector dimensions based on the provider:
- Ollama (nomic-embed-text): 768 dimensions
- OpenAI (text-embedding-3-small): 1536 dimensions
Acknowledgments
This project is a fork of qpd-v/mcp-ragdocs, originally developed by qpd-v. The original project provided the foundation for this implementation.
Special thanks to the original creator, qpd-v, for their innovative work on the initial version of this MCP server. This fork has been enhanced with additional features and improvements by Rahul Retnan.
Troubleshooting
Server Not Starting (Port Conflict)
If the MCP server fails to start due to a port conflict, follow these steps:
- Identify and kill the process using port 3030:
npx kill-port 3030
-
Restart the MCP server
-
If the issue persists, check for other processes using the port:
lsof -i :3030
- You can also change the default port in the configuration if needed
Recommended Servers
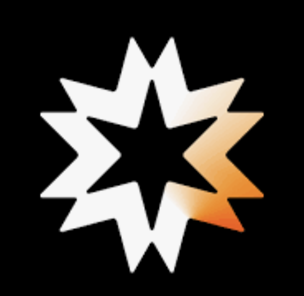
E2B
Using MCP to run code via e2b.
Exa Search
A Model Context Protocol (MCP) server lets AI assistants like Claude use the Exa AI Search API for web searches. This setup allows AI models to get real-time web information in a safe and controlled way.
Exa MCP
A Model Context Protocol server that enables AI assistants like Claude to perform real-time web searches using the Exa AI Search API in a safe and controlled manner.
Perplexity Chat MCP Server
MCP Server for the Perplexity API.
Web Research Server
A Model Context Protocol server that enables Claude to perform web research by integrating Google search, extracting webpage content, and capturing screenshots.
PubMedSearch
A Model Content Protocol server that provides tools to search and retrieve academic papers from PubMed database.
Aindreyway Codex Keeper
Serves as a guardian of development knowledge, providing AI assistants with curated access to latest documentation and best practices.
Perplexity Deep Research
A server that allows AI assistants to perform web searches using Perplexity's sonar-deep-research model with citation support.

Docx Document Processing Service
A powerful Word document processing service based on FastMCP, enabling AI assistants to create, edit, and manage docx files with full formatting support. Preserves original styles when editing content.
Web Research Server
MCP web research server (give Claude real-time info from the web) - oneshot-engineering/mcp-webresearch