Memory Service
Provides semantic memory and persistent storage for Claude, leveraging ChromaDB and sentence transformers for enhanced search and retrieval capabilities.
doobidoo
Tools
store_memory
Store new information with optional tags
retrieve_memory
Find relevant memories based on query
search_by_tag
Search memories by tags
README
MCP Memory Service
An MCP server providing semantic memory and persistent storage capabilities for Claude Desktop using ChromaDB and sentence transformers. This service enables long-term memory storage with semantic search capabilities, making it ideal for maintaining context across conversations and instances.
<img width="240" alt="grafik" src="https://github.com/user-attachments/assets/eab1f341-ca54-445c-905e-273cd9e89555" /> <a href="https://glama.ai/mcp/servers/bzvl3lz34o"><img width="380" height="200" src="https://glama.ai/mcp/servers/bzvl3lz34o/badge" alt="Memory Service MCP server" /></a>
Features
- Semantic search using sentence transformers
- Natural language time-based recall (e.g., "last week", "yesterday morning")
- Tag-based memory retrieval system
- Persistent storage using ChromaDB
- Automatic database backups
- Memory optimization tools
- Exact match retrieval
- Debug mode for similarity analysis
- Database health monitoring
- Duplicate detection and cleanup
- Customizable embedding model
- Cross-platform compatibility (Apple Silicon, Intel, Windows, Linux)
- Hardware-aware optimizations for different environments
- Graceful fallbacks for limited hardware resources
Quick Start
For the fastest way to get started:
# Install UV if not already installed
pip install uv
# Clone and install
git clone https://github.com/doobidoo/mcp-memory-service.git
cd mcp-memory-service
uv venv
source .venv/bin/activate # On Windows: .venv\Scripts\activate
uv pip install -r requirements.txt
uv pip install -e .
# Run the service
uv run memory
Docker and Smithery Integration
Docker Usage
The service can be run in a Docker container for better isolation and deployment:
# Build the Docker image
docker build -t mcp-memory-service .
# Run the container
# Note: On macOS, paths must be within Docker's allowed file sharing locations
# Default allowed locations include:
# - /Users
# - /Volumes
# - /private
# - /tmp
# - /var/folders
# Example with proper macOS paths:
docker run -it \
-v $HOME/mcp-memory/chroma_db:/app/chroma_db \
-v $HOME/mcp-memory/backups:/app/backups \
mcp-memory-service
# For production use, you might want to run it in detached mode:
docker run -d \
-v $HOME/mcp-memory/chroma_db:/app/chroma_db \
-v $HOME/mcp-memory/backups:/app/backups \
--name mcp-memory \
mcp-memory-service
To configure Docker's file sharing on macOS:
- Open Docker Desktop
- Go to Settings (Preferences)
- Navigate to Resources -> File Sharing
- Add any additional paths you need to share
- Click "Apply & Restart"
Smithery Integration
The service is configured for Smithery integration through smithery.yaml
. This configuration enables stdio-based communication with MCP clients like Claude Desktop.
To use with Smithery:
- Ensure your
claude_desktop_config.json
points to the correct paths:
{
"memory": {
"command": "docker",
"args": [
"run",
"-i",
"--rm",
"-v", "$HOME/mcp-memory/chroma_db:/app/chroma_db",
"-v", "$HOME/mcp-memory/backups:/app/backups",
"mcp-memory-service"
],
"env": {
"MCP_MEMORY_CHROMA_PATH": "/app/chroma_db",
"MCP_MEMORY_BACKUPS_PATH": "/app/backups"
}
}
}
- The
smithery.yaml
configuration handles stdio communication and environment setup automatically.
Testing with Claude Desktop
To verify your Docker-based memory service is working correctly with Claude Desktop:
- Build the Docker image with
docker build -t mcp-memory-service .
- Create the necessary directories for persistent storage:
mkdir -p $HOME/mcp-memory/chroma_db $HOME/mcp-memory/backups
- Update your Claude Desktop configuration file:
- On macOS:
~/Library/Application Support/Claude/claude_desktop_config.json
- On Windows:
%APPDATA%\Claude\claude_desktop_config.json
- On Linux:
~/.config/Claude/claude_desktop_config.json
- On macOS:
- Restart Claude Desktop
- When Claude starts up, you should see the memory service initialize with a message:
MCP Memory Service initialization completed
- Test the memory feature:
- Ask Claude to remember something: "Please remember that my favorite color is blue"
- Later in the conversation or in a new conversation, ask: "What is my favorite color?"
- Claude should retrieve the information from the memory service
If you experience any issues:
- Check the Claude Desktop console for error messages
- Verify Docker has the necessary permissions to access the mounted directories
- Ensure the Docker container is running with the correct parameters
- Try running the container manually to see any error output
For detailed installation instructions, platform-specific guides, and troubleshooting, see our documentation:
- Installation Guide - Comprehensive installation instructions for all platforms
- Troubleshooting Guide - Solutions for common issues
- Technical Documentation - Detailed technical procedures and specifications
- Scripts Documentation - Overview of available scripts and their usage
Configuration
Standard Configuration (Recommended)
Add the following to your claude_desktop_config.json
file to use UV (recommended for best performance):
{
"memory": {
"command": "uv",
"args": [
"--directory",
"your_mcp_memory_service_directory", // e.g., "C:\\REPOSITORIES\\mcp-memory-service"
"run",
"memory"
],
"env": {
"MCP_MEMORY_CHROMA_PATH": "your_chroma_db_path", // e.g., "C:\\Users\\John.Doe\\AppData\\Local\\mcp-memory\\chroma_db"
"MCP_MEMORY_BACKUPS_PATH": "your_backups_path" // e.g., "C:\\Users\\John.Doe\\AppData\\Local\\mcp-memory\\backups"
}
}
}
Windows-Specific Configuration (Recommended)
For Windows users, we recommend using the wrapper script to ensure PyTorch is properly installed. See our Windows Setup Guide for detailed instructions.
{
"memory": {
"command": "python",
"args": [
"C:\\path\\to\\mcp-memory-service\\memory_wrapper.py"
],
"env": {
"MCP_MEMORY_CHROMA_PATH": "C:\\Users\\YourUsername\\AppData\\Local\\mcp-memory\\chroma_db",
"MCP_MEMORY_BACKUPS_PATH": "C:\\Users\\YourUsername\\AppData\\Local\\mcp-memory\\backups"
}
}
}
The wrapper script will:
- Check if PyTorch is installed and properly configured
- Install PyTorch with the correct index URL if needed
- Run the memory server with the appropriate configuration
Hardware Compatibility
Platform | Architecture | Accelerator | Status |
---|---|---|---|
macOS | Apple Silicon (M1/M2/M3) | MPS | ✅ Fully supported |
macOS | Apple Silicon under Rosetta 2 | CPU | ✅ Supported with fallbacks |
macOS | Intel | CPU | ✅ Fully supported |
Windows | x86_64 | CUDA | ✅ Fully supported |
Windows | x86_64 | DirectML | ✅ Supported |
Windows | x86_64 | CPU | ✅ Supported with fallbacks |
Linux | x86_64 | CUDA | ✅ Fully supported |
Linux | x86_64 | ROCm | ✅ Supported |
Linux | x86_64 | CPU | ✅ Supported with fallbacks |
Linux | ARM64 | CPU | ✅ Supported with fallbacks |
Memory Operations
The memory service provides the following operations through the MCP server:
Core Memory Operations
store_memory
- Store new information with optional tagsretrieve_memory
- Perform semantic search for relevant memoriesrecall_memory
- Retrieve memories using natural language time expressionssearch_by_tag
- Find memories using specific tagsexact_match_retrieve
- Find memories with exact content matchdebug_retrieve
- Retrieve memories with similarity scores
For detailed information about tag storage and management, see our Tag Storage Documentation.
Database Management
create_backup
- Create database backupget_stats
- Get memory statisticsoptimize_db
- Optimize database performancecheck_database_health
- Get database health metricscheck_embedding_model
- Verify model status
Memory Management
delete_memory
- Delete specific memory by hashdelete_by_tag
- Delete all memories with specific tagcleanup_duplicates
- Remove duplicate entries
Configuration Options
Configure through environment variables:
CHROMA_DB_PATH: Path to ChromaDB storage
BACKUP_PATH: Path for backups
AUTO_BACKUP_INTERVAL: Backup interval in hours (default: 24)
MAX_MEMORIES_BEFORE_OPTIMIZE: Threshold for auto-optimization (default: 10000)
SIMILARITY_THRESHOLD: Default similarity threshold (default: 0.7)
MAX_RESULTS_PER_QUERY: Maximum results per query (default: 10)
BACKUP_RETENTION_DAYS: Number of days to keep backups (default: 7)
LOG_LEVEL: Logging level (default: INFO)
# Hardware-specific environment variables
PYTORCH_ENABLE_MPS_FALLBACK: Enable MPS fallback for Apple Silicon (default: 1)
MCP_MEMORY_USE_ONNX: Use ONNX Runtime for CPU-only deployments (default: 0)
MCP_MEMORY_USE_DIRECTML: Use DirectML for Windows acceleration (default: 0)
MCP_MEMORY_MODEL_NAME: Override the default embedding model
MCP_MEMORY_BATCH_SIZE: Override the default batch size
Getting Help
If you encounter any issues:
- Check our Troubleshooting Guide
- Review the Installation Guide
- For Windows-specific issues, see our Windows Setup Guide
- Contact the developer via Telegram: t.me/doobeedoo
Project Structure
mcp-memory-service/
├── src/mcp_memory_service/ # Core package code
│ ├── __init__.py
│ ├── config.py # Configuration utilities
│ ├── models/ # Data models
│ ├── storage/ # Storage implementations
│ ├── utils/ # Utility functions
│ └── server.py # Main MCP server
├── scripts/ # Helper scripts
│ ├── convert_to_uv.py # Script to migrate to UV
│ └── install_uv.py # UV installation helper
├── .uv/ # UV configuration
├── memory_wrapper.py # Windows wrapper script
├── memory_wrapper_uv.py # UV-based wrapper script
├── uv_wrapper.py # UV wrapper script
├── install.py # Enhanced installation script
└── tests/ # Test suite
Development Guidelines
- Python 3.10+ with type hints
- Use dataclasses for models
- Triple-quoted docstrings for modules and functions
- Async/await pattern for all I/O operations
- Follow PEP 8 style guidelines
- Include tests for new features
License
MIT License - See LICENSE file for details
Acknowledgments
- ChromaDB team for the vector database
- Sentence Transformers project for embedding models
- MCP project for the protocol specification
Contact
Recommended Servers
playwright-mcp
A Model Context Protocol server that enables LLMs to interact with web pages through structured accessibility snapshots without requiring vision models or screenshots.
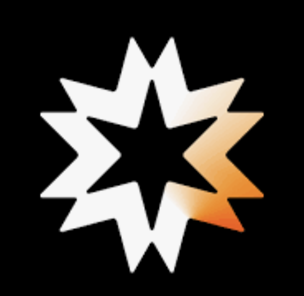
E2B
Using MCP to run code via e2b.
Neon Database
MCP server for interacting with Neon Management API and databases
Exa Search
A Model Context Protocol (MCP) server lets AI assistants like Claude use the Exa AI Search API for web searches. This setup allows AI models to get real-time web information in a safe and controlled way.
Qdrant Server
This repository is an example of how to create a MCP server for Qdrant, a vector search engine.
Mult Fetch MCP Server
A versatile MCP-compliant web content fetching tool that supports multiple modes (browser/node), formats (HTML/JSON/Markdown/Text), and intelligent proxy detection, with bilingual interface (English/Chinese).
AIO-MCP Server
🚀 All-in-one MCP server with AI search, RAG, and multi-service integrations (GitLab/Jira/Confluence/YouTube) for AI-enhanced development workflows. Folk from
Persistent Knowledge Graph
An implementation of persistent memory for Claude using a local knowledge graph, allowing the AI to remember information about users across conversations with customizable storage location.
React MCP
react-mcp integrates with Claude Desktop, enabling the creation and modification of React apps based on user prompts
Atlassian Integration
Model Context Protocol (MCP) server for Atlassian Cloud products (Confluence and Jira). This integration is designed specifically for Atlassian Cloud instances and does not support Atlassian Server or Data Center deployments.