Axiom MCP Server
An npx-compatible port of @Axiom's mcp-server-axiom
ThetaBird
Tools
queryApl
# Instructions 1. Query Axiom datasets using Axiom Processing Language (APL). The query must be a valid APL query string. 2. ALWAYS get the schema of the dataset before running queries rather than guessing. You can do this by getting a single event and projecting all fields. 3. Keep in mind that there's a maximum row limit of 65000 rows per query. 4. Prefer aggregations over non aggregating queries when possible to reduce the amount of data returned. 5. Be selective in what you project in each query (unless otherwise needed, like for discovering the schema). It's expensive to project all fields. 6. ALWAYS restrict the time range of the query to the smallest possible range that meets your needs. This will reduce the amount of data scanned and improve query performance. 7. NEVER guess the schema of the dataset. If you don't where something is, use search first to find in which fields it appears. # Examples Basic: - Filter: ['logs'] | where ['severity'] == "error" or ['duration'] > 500ms - Time range: ['logs'] | where ['_time'] > ago(2h) and ['_time'] < now() - Project rename: ['logs'] | project-rename responseTime=['duration'], path=['url'] Aggregations: - Count by: ['logs'] | summarize count() by bin(['_time'], 5m), ['status'] - Multiple aggs: ['logs'] | summarize count(), avg(['duration']), max(['duration']), p95=percentile(['duration'], 95) by ['endpoint'] - Dimensional: ['logs'] | summarize dimensional_analysis(['isError'], pack_array(['endpoint'], ['status'])) - Histograms: ['logs'] | summarize histogram(['responseTime'], 100) by ['endpoint'] - Distinct: ['logs'] | summarize dcount(['userId']) by bin_auto(['_time']) Search & Parse: - Search all: search "error" or "exception" - Parse logs: ['logs'] | parse-kv ['message'] as (duration:long, error:string) with (pair_delimiter=",") - Regex extract: ['logs'] | extend errorCode = extract("error code ([0-9]+)", 1, ['message']) - Contains ops: ['logs'] | where ['message'] contains_cs "ERROR" or ['message'] startswith "FATAL" Data Shaping: - Extend & Calculate: ['logs'] | extend duration_s = ['duration']/1000, success = ['status'] < 400 - Dynamic: ['logs'] | extend props = parse_json(['properties']) | where ['props.level'] == "error" - Pack/Unpack: ['logs'] | extend fields = pack("status", ['status'], "duration", ['duration']) - Arrays: ['logs'] | where ['url'] in ("login", "logout", "home") | where array_length(['tags']) > 0 Advanced: - Make series: ['metrics'] | make-series avg(['cpu']) default=0 on ['_time'] step 1m by ['host'] - Join: ['errors'] | join kind=inner (['users'] | project ['userId'], ['email']) on ['userId'] - Union: union ['logs-app*'] | where ['severity'] == "error" - Fork: ['logs'] | fork (where ['status'] >= 500 | as errors) (where ['status'] < 300 | as success) - Case: ['logs'] | extend level = case(['status'] >= 500, "error", ['status'] >= 400, "warn", "info") Time Operations: - Bin & Range: ['logs'] | where ['_time'] between(datetime(2024-01-01)..now()) - Multiple time bins: ['logs'] | summarize count() by bin(['_time'], 1h), bin(['_time'], 1d) - Time shifts: ['logs'] | extend prev_hour = ['_time'] - 1h String Operations: - String funcs: ['logs'] | extend domain = tolower(extract("://([^/]+)", 1, ['url'])) - Concat: ['logs'] | extend full_msg = strcat(['level'], ": ", ['message']) - Replace: ['logs'] | extend clean_msg = replace_regex("(password=)[^&]*", "\1***", ['message']) Common Patterns: - Error analysis: ['logs'] | where ['severity'] == "error" | summarize error_count=count() by ['error_code'], ['service'] - Status codes: ['logs'] | summarize requests=count() by ['status'], bin_auto(['_time']) | where ['status'] >= 500 - Latency tracking: ['logs'] | summarize p50=percentile(['duration'], 50), p90=percentile(['duration'], 90) by ['endpoint'] - User activity: ['logs'] | summarize user_actions=count() by ['userId'], ['action'], bin(['_time'], 1h)
listDatasets
List all available Axiom datasets
getDatasetInfoAndSchema
Get dataset info and schema
README
MCP Server for Axiom
A JavaScript port of the official Axiom MCP server that enables AI agents to query data using Axiom Processing Language (APL).
<a href="https://glama.ai/mcp/servers/8hxxw8uenu"> <img width="380" height="200" src="https://glama.ai/mcp/servers/8hxxw8uenu/badge" /> </a>
This implementation provides the same functionality as the original Go version but packaged as an npm module for easier integration with Node.js environments.
Installation & Usage
MCP Configuration
You can run this MCP server directly using npx. Add the following configuration to your MCP configuration file:
{
"axiom": {
"command": "npx",
"args": ["-y", "mcp-server-axiom"],
"env": {
"AXIOM_TOKEN": "<AXIOM_TOKEN_HERE>",
"AXIOM_URL": "https://api.axiom.co",
"AXIOM_ORG_ID": "<AXIOM_ORG_ID_HERE>"
}
}
}
Local Development & Testing
Installation
npm install -g mcp-server-axiom
Environment Variables
The server can be configured using environment variables:
AXIOM_TOKEN
(required): Your Axiom API tokenAXIOM_ORG_ID
(required): Your Axiom organization IDAXIOM_URL
(optional): Custom Axiom API URL (defaults to https://api.axiom.co)AXIOM_QUERY_RATE
(optional): Queries per second limit (default: 1)AXIOM_QUERY_BURST
(optional): Query burst capacity (default: 1)AXIOM_DATASETS_RATE
(optional): Dataset list operations per second (default: 1)AXIOM_DATASETS_BURST
(optional): Dataset list burst capacity (default: 1)PORT
(optional): Server port (default: 3000)
Running the Server Locally
- Using environment variables:
export AXIOM_TOKEN=your_token
mcp-server-axiom
- Using a config file:
mcp-server-axiom config.json
Example config.json:
{
"token": "your_token",
"url": "https://custom.axiom.co",
"orgId": "your_org_id",
"queryRate": 2,
"queryBurst": 5,
"datasetsRate": 1,
"datasetsBurst": 2
}
API Endpoints
GET /
: Get server implementation infoGET /tools
: List available toolsPOST /tools/:name/call
: Call a specific tool- Available tools:
queryApl
: Execute APL querieslistDatasets
: List available datasets
- Available tools:
Example Tool Calls
- Query APL:
curl -X POST http://localhost:3000/tools/queryApl/call \
-H "Content-Type: application/json" \
-d '{
"arguments": {
"query": "['logs'] | where ['severity'] == \"error\" | limit 10"
}
}'
- List Datasets:
curl -X POST http://localhost:3000/tools/listDatasets/call \
-H "Content-Type: application/json" \
-d '{
"arguments": {}
}'
License
MIT
Recommended Servers
playwright-mcp
A Model Context Protocol server that enables LLMs to interact with web pages through structured accessibility snapshots without requiring vision models or screenshots.
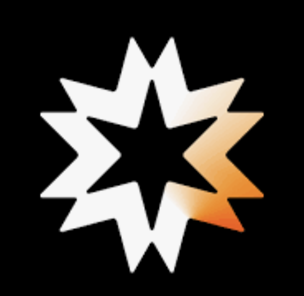
E2B
Using MCP to run code via e2b.
Neon Database
MCP server for interacting with Neon Management API and databases
Exa Search
A Model Context Protocol (MCP) server lets AI assistants like Claude use the Exa AI Search API for web searches. This setup allows AI models to get real-time web information in a safe and controlled way.
Qdrant Server
This repository is an example of how to create a MCP server for Qdrant, a vector search engine.
AIO-MCP Server
🚀 All-in-one MCP server with AI search, RAG, and multi-service integrations (GitLab/Jira/Confluence/YouTube) for AI-enhanced development workflows. Folk from
Persistent Knowledge Graph
An implementation of persistent memory for Claude using a local knowledge graph, allowing the AI to remember information about users across conversations with customizable storage location.
Hyperbrowser MCP Server
Welcome to Hyperbrowser, the Internet for AI. Hyperbrowser is the next-generation platform empowering AI agents and enabling effortless, scalable browser automation. Built specifically for AI developers, it eliminates the headaches of local infrastructure and performance bottlenecks, allowing you to
React MCP
react-mcp integrates with Claude Desktop, enabling the creation and modification of React apps based on user prompts
Atlassian Integration
Model Context Protocol (MCP) server for Atlassian Cloud products (Confluence and Jira). This integration is designed specifically for Atlassian Cloud instances and does not support Atlassian Server or Data Center deployments.